Synthetic Skin Cancer Detection Dataset
Patient Health Records & Digital Health
Related Searches
Trusted By
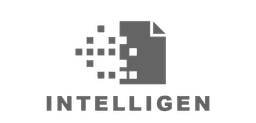
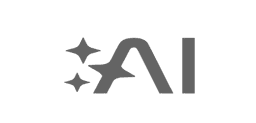
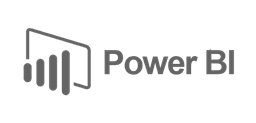
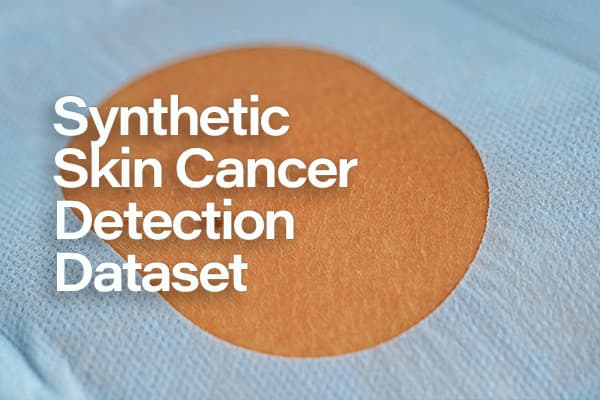
"No reviews yet"
£199.99
About
The Synthetic Skin Cancer Detection Dataset is designed for educational and research purposes to analyze factors associated with skin cancer types, their diagnosis, and treatment options. The dataset includes anonymized, synthetic data on various clinical and demographic factors for individuals diagnosed with different types of skin cancer.
Dataset Features
- Participant ID: Unique identifier for each participant.
- MEL: Melanoma (Yes/No).
- NV: Melanocytic Nevus (Yes/No).
- BCC: Basal Cell Carcinoma (Yes/No).
- AKIEC: Actinic Keratoses (Yes/No).
- BKL: Benign Keratosis (Yes/No).
- DF: Dermatofibroma (Yes/No).
- VASC: Vascular Lesions (Yes/No).
Distribution
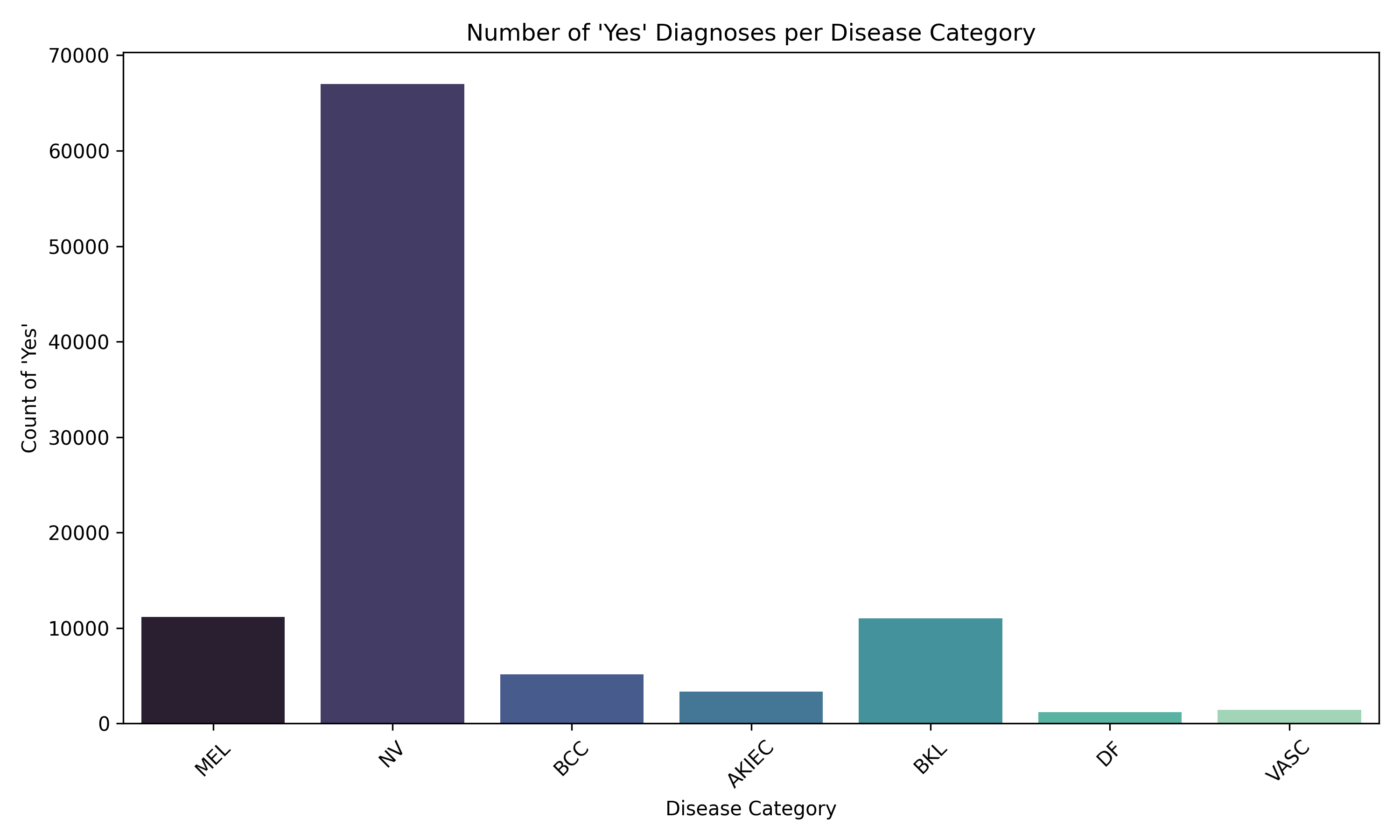
Usage
This dataset can be used for the following applications:
- Cancer Research: Investigate the relationship between various demographic, clinical, and tumour-type factors with the presence of skin cancer.
- Predictive Modeling: Build machine learning models to predict the presence or progression of different skin cancer types based on participant data.
- Clinical Research: Study the impact of various factors like lesion type, treatment options, and demographic data on skin cancer prognosis.
- Educational Purposes: Provide a dataset for students and researchers in oncology, dermatology, and medical data science to analyze skin cancer detection and treatment patterns.
Coverage
This synthetic dataset is fully anonymized and complies with data privacy standards. It includes a broad set of factors to support diverse research and analysis in the oncology and medical domains, particularly in dermatology.
License
CC0 (Public Domain)
Who Can Use It
- Cancer Researchers: To explore correlations between clinical factors, lesion types, and treatment outcomes in skin cancer.
- Dermatologists and Healthcare Providers: To analyze how skin lesions and treatments affect cancer progression and recovery.
- Data Scientists and Machine Learning Practitioners: To develop predictive models for skin cancer diagnosis, classification, and prognosis.
- Educators and Students: As a resource for studying skin cancer analytics and medical data science.