Synthetic Ovarian Cancer Prediction Dataset
Patient Health Records & Digital Health
Related Searches
Trusted By
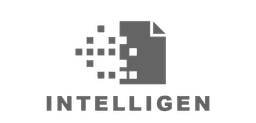
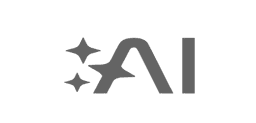
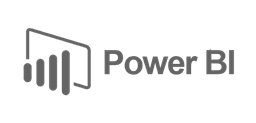
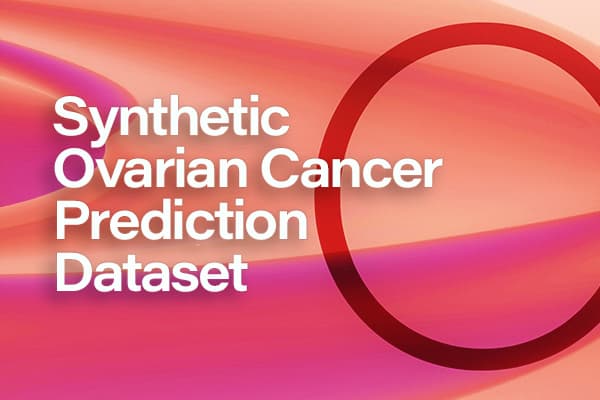
"No reviews yet"
£199.99
About
The Synthetic Ovarian Cancer Prediction Dataset is created for educational and research purposes, particularly for the exploration and development of machine learning models to predict ovarian cancer presence and progression. The dataset includes anonymized, synthetic clinical and laboratory data for 100,000 subjects, simulating real-world patterns of ovarian cancer indicators.
Dataset Features
The dataset includes 51 features representing a wide range of blood biomarkers, demographics, and diagnostic markers commonly associated with ovarian cancer. These include:
- SUBJECT_ID: Unique identifier for each patient.
- Age: Age of the patient (in years).
- Menopause: Menopausal status (encoded as integers).
- AFP, CA125, CA19-9, CA72-4, HE4, CEA: Tumor markers relevant to cancer diagnostics.
- Various Blood Markers: Including ALB, AST, ALT, ALP, BUN, CREA, TP, TBIL, DBIL, IBIL, GGT, GLU, and others.
- Electrolytes and Ions: Such as Na, K, Ca, Mg, CL, PHOS, CO2CP.
- Hematological Measures: Including HGB, HCT, RBC, WBC subtypes (NEU, LYM, MONO, EO, BASO), PLT, RDW, MCH, MCV, MPV, PDW, and PCT.
- TYPE: Target variable indicating cancer classification (encoded as integers).
Distribution
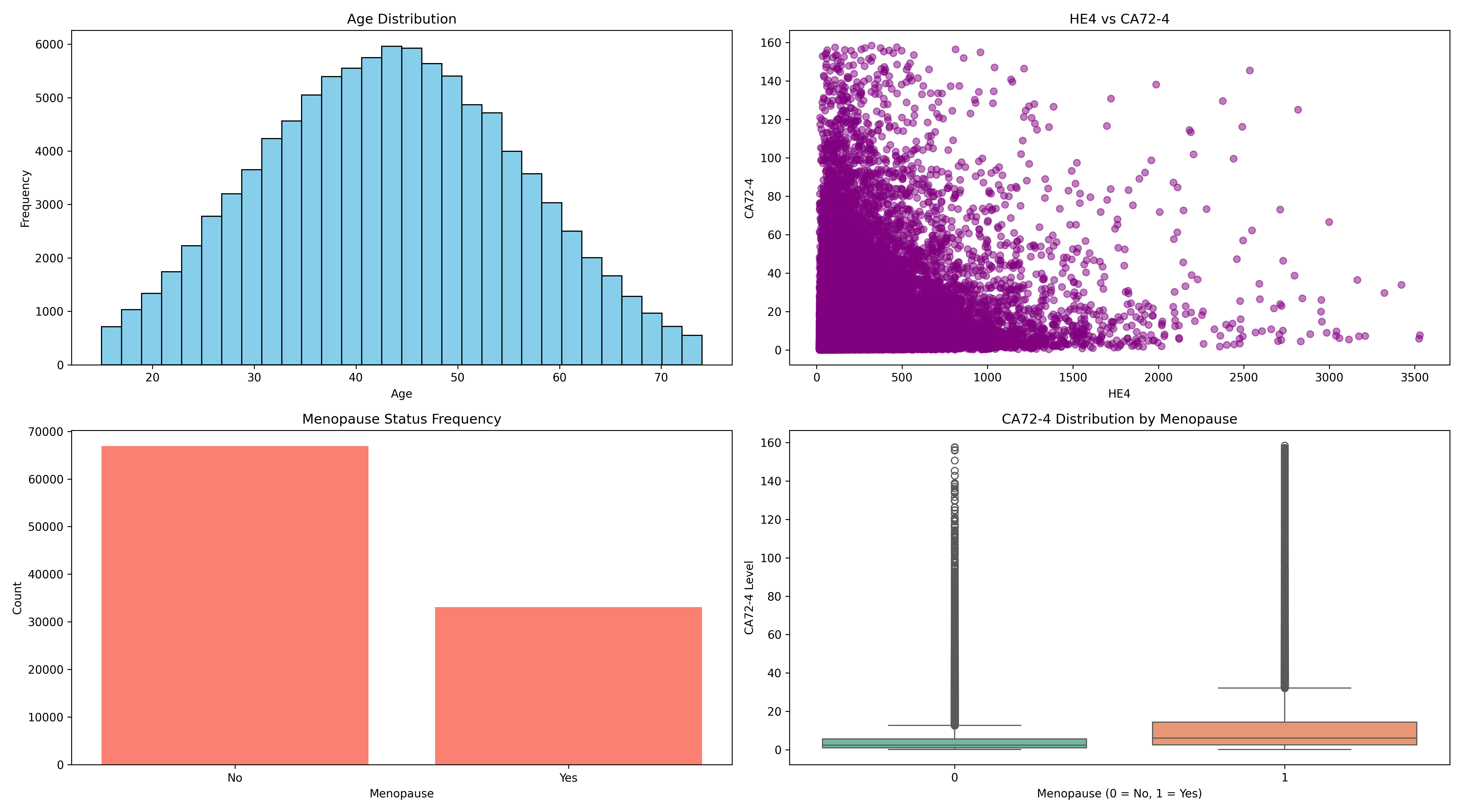
Usage
This dataset can be used for the following applications:
- Cancer Research: Explore associations between biomarkers and ovarian cancer diagnosis or prognosis.
- Predictive Modeling: Develop machine learning or statistical models to classify or predict ovarian cancer types based on lab results and demographic data.
- Clinical Research: Study the diagnostic value of various tumor markers in ovarian cancer.
- Educational Purposes: Serve as a resource for training students and professionals in data science, bioinformatics, and healthcare analytics.
Coverage
The dataset is fully synthetic and anonymized, adhering to privacy standards and suitable for open-access educational and research purposes. It captures realistic data distributions for key clinical metrics involved in ovarian cancer detection and monitoring.
License
CC0 (Public Domain)
Who Can Use It
- Medical Researchers and Oncologists: To identify biomarker trends and diagnostic criteria for ovarian cancer.
- Data Scientists and Machine Learning Engineers: To build and evaluate predictive models on medical data.
- Healthcare Educators and Students: For academic instruction, project work, and data analysis practice in clinical research settings.